In the dynamic landscape of retail, advertising plays a pivotal role in driving customer engagement, brand visibility, and ultimately, sales. As the industry evolves, leveraging advanced technologies like Artificial Intelligence (AI) has become crucial for retailers to stay competitive.
In part 5 of the series, Retail Mashup discussed AI in retail marketing. This part goes further by focusing on how AI can play a role in ad networks in helping brands connect further with consumers.
Table of Contents
What is an Ad Network?
An ad network is a platform that connects advertisers with publishers who want to host advertisements. Ad networks serve as intermediaries, facilitating the buying and selling of ad inventory. Advertisers use ad networks to reach a wider audience by displaying their ads on multiple websites or apps within the network.
Publishers like Google, on the other hand, use ad networks to monetize their websites or apps by displaying ads and earning revenue based on ad impressions or clicks. Ad networks typically use targeting criteria such as demographics, interests, and browsing behavior to deliver relevant ads to users.
The Rise of AI in Ad Networks
AI has transformed traditional ad networks into intelligent systems capable of delivering personalized, targeted advertisements to consumers. By leveraging machine learning algorithms, these networks analyze vast amounts of data to understand consumer behavior, preferences, and trends. This enables retailers to create highly targeted campaigns that resonate with their target audience, leading to improved conversion rates and return on investment (ROI).
Here’s a brief timeline highlighting key milestones in the integration of AI into ad networks:
- Early 2000s: The use of AI in ad networks began with basic algorithms for ad targeting and optimization. These early systems laid the foundation for more sophisticated AI applications in later years.
- Mid-2000s: Google introduced its AdSense program in 2003, which used AI algorithms to match ads with relevant content on websites. This marked a significant advancement in contextual advertising powered by AI.
- Late 2000s to Early 2010s: The introduction of real-time bidding (RTB) platforms in the late 2000s and early 2010s led to the integration of AI for ad targeting and bidding optimization. Ad networks began using machine learning algorithms to analyze vast amounts of data in real time to improve ad performance.
- 2010s: The 2010s saw a rapid expansion of AI applications in ad networks, driven by advancements in machine learning and data processing technologies. Companies like Google, Facebook, and Amazon invested heavily in AI to enhance ad targeting, personalization, and campaign optimization.
- Mid-2010s: The mid-2010s witnessed the emergence of programmatic advertising, which relies heavily on AI for automated ad buying and selling. AI-powered programmatic platforms revolutionized the ad buying process, making it more efficient and cost-effective.
- Late 2010s to Present: AI continues to play a central role in ad networks, with a focus on advanced targeting, dynamic ad creative optimization, and cross-device targeting. AI-powered ad networks are now capable of delivering highly personalized and targeted ads to users across various channels and devices.
Statistics on AI in Retail Advertising
The adoption of AI in retail marketing is on the rise, driven by the need for more efficient and effective marketing strategies. According to a report by Grand View Research, AI-powered retail marketing is estimated to reach US$40.74 billion by 2030, expanding at a Compounded Annual Growth Rate (CAGR) of 23.9% from 2022 to 2030. This growth is fuelled by the increasing demand for personalized shopping experiences and the need for retailers to optimize and isolate their marketing efforts to specific demographics.
AI-powered retail marketing size by 2030
0USD$ (millions)
Brands Using Intelligent Ad Networks
Quite a few technology companies have embraced AI-powered ad networks to enhance their advertising strategies. Two notable examples are Amazon and Walmart.
Amazon uses AI algorithms to analyze customer data and behavior to deliver personalized product recommendations and targeted advertisements. This has helped Amazon significantly improve its advertising revenue and drive sales on its platform.
Walmart uses AI to analyze customer data and optimize its ad campaigns across various channels. By leveraging AI-powered ad networks, Walmart has been able to improve its targeting accuracy and deliver more relevant ads both digitally (online) and physical (at stores) to its customers, leading to higher conversion rates and ROI.
Beyond the two giant retailers, other examples that created an ad network eco-system include:
- Google: Google is a major player in the ad network space through its Google Ads platform. Google uses AI to improve ad targeting, optimize bids, and enhance ad placements across its network of websites and apps. Google’s AI algorithms analyze user behavior, search history, and other data points to deliver more relevant and personalized ads to users.
- Facebook: Facebook’s ad network, including Facebook Ads and Instagram Ads, uses AI to target ads based on user interests, demographics, and behavior. Facebook’s AI algorithms continuously learn from user interactions to improve ad targeting and performance.
- Microsoft: Microsoft’s ad network, powered by its Bing search engine, Open AI and Microsoft Advertising platform, uses AI to improve ad targeting, ad copy optimization, and bid management. Microsoft’s AI capabilities also extend to its LinkedIn platform, where it helps advertisers target professionals based on their profiles and behavior.
- The Trade Desk: The Trade Desk is a programmatic advertising platform that uses AI for ad buying, audience targeting, and campaign optimization, making it a popular choice among advertisers.
- CVS: The CVS ad network, also known as CVS Media Exchange, is a digital advertising platform operated by CVS Health, one of the largest retail pharmacy chains in the United States. The network allows advertisers to reach CVS customers through targeted digital ads across various channels, including the CVS website, mobile app, email, and in-store displays. With over 9,900 retail locations across the country and millions of customers, CVS offers advertisers a significant reach and the opportunity to engage with a highly valuable audience.
Pros and Cons of AI-Powered Ad Networks in Retail
Before getting into the AI-Powered ad networks, brands should consider the following pros and cons:
Pros:
- Personalization: AI enables retailers to create personalized ad campaigns based on individual customer preferences and behavior, leading to higher engagement and conversion rates.
- Targeting Accuracy: AI algorithms analyze vast amounts of data to identify the most relevant audience segments for a particular campaign, ensuring that ads are shown to the right people at the right time.
- Optimization: AI continuously optimizes ad campaigns based on real-time data, allowing retailers to maximize their ROI and drive more sales.
- Cost-Effectiveness: By targeting the right audience segments, AI-powered ad networks help retailers reduce wasted ad spend and improve the efficiency of their marketing efforts.
- Competitive Advantage: Retailers that leverage AI in their advertising strategies gain a competitive edge by delivering more relevant and engaging ads to their customers.
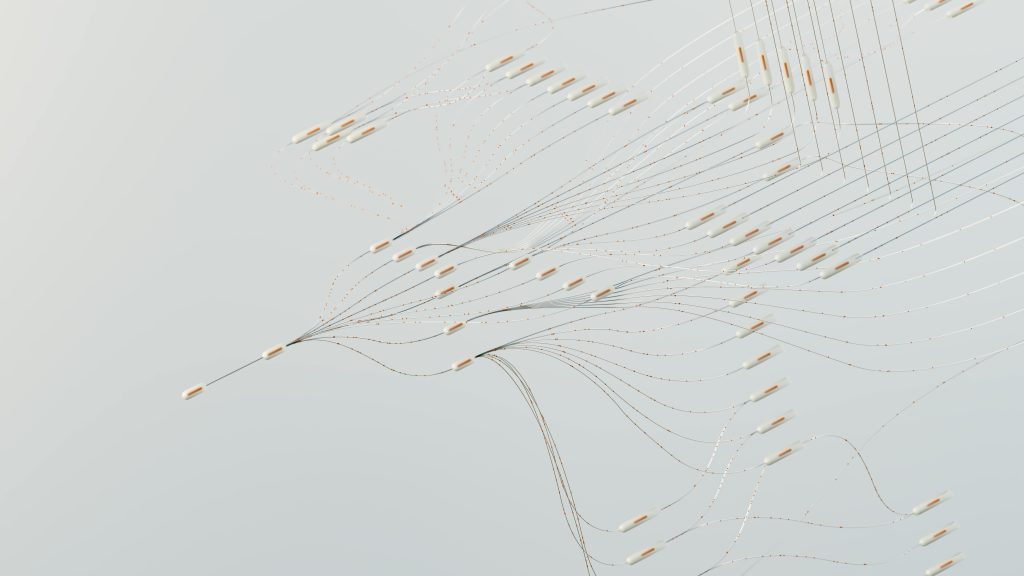
Cons:
- Cost: Implementing and managing AI-powered ad campaigns can be expensive, especially for small and medium-sized retailers with limited budgets. AI technologies require ongoing investment in infrastructure, tools, and talent.
- Complexity: AI-powered ad networks can be complex to set up and manage, requiring expertise in data analysis, machine learning, and digital marketing. Retailers may need to invest in training or hiring skilled professionals to effectively leverage AI in their advertising efforts.
- Data Privacy Concerns: AI-powered ad networks rely on collecting and analyzing large amounts of customer data, raising concerns about data privacy and security. Retailers must comply with regulations such as GDPR and CCPA to protect customer data and ensure ethical use of AI.
- Dependency on Data Quality: AI algorithms depend on high-quality, accurate data to deliver meaningful insights and predictions. Retailers need to ensure that their data is clean, up-to-date, and relevant for AI-powered ad campaigns to be effective.
- Risk of Bias: AI algorithms can inadvertently perpetuate bias if they are trained on biased data or programmed with biased instructions. Retailers must carefully monitor and audit their AI systems to mitigate the risk of bias in their advertising campaigns.
Metrics to Measure Success
Measuring the effectiveness of using AI in ad networks involves tracking various metrics that indicate the performance and impact of AI-powered advertising campaigns.
Here are some key metrics to consider:
- Click-Through Rate (CTR): CTR measures the percentage of people who click on an ad after seeing it. A high CTR indicates that the ad is engaging and relevant to the audience. Goal: Higher is better.
- Conversion Rate: The conversion rate measures the percentage of people who take a desired action after clicking on an ad, such as making a purchase or signing up for a newsletter. AI can help optimize campaigns to improve conversion rates. Goal: Higher is better.
- Return on Ad Spend (ROAS): ROAS measures the revenue generated for every dollar spent on advertising (Revenue from ads divide by Cost from ads). AI can help maximize ROAS by targeting the right audience segments and optimizing ad campaigns for better performance. Goal: Higher is better.
- Cost per Acquisition (CPA): CPA measures the cost of acquiring a customer through advertising. AI can help reduce CPA by improving targeting and optimizing ad spend. Goal: Lower is better.
- Engagement Metrics: Metrics such as time spent on site (time-based), pages per visit, and bounce rate (%) can indicate the effectiveness of ads in engaging the audience and driving them to take action. Goal: Higher is better except for bounce rate.
- Audience Insights: AI can provide valuable insights into audience behavior, preferences, and trends, which can help tailor ad campaigns for better performance. Goal: Higher engagement is preferred and may lead to higher sales
- Ad Placement and Performance: AI can analyze the performance of ads on different platforms and placements to determine which ones are most effective for reaching the target audience. Goal: Similar to conversation rate
- Brand Lift: AI can measure the impact of ads on brand awareness, perception, and recall, providing insights into the overall effectiveness of the advertising campaign. Goal: Higher positive product or brand awareness is preferred
- Incremental Sales: AI can help measure the incremental sales generated by advertising campaigns, taking into account the impact of ads on consumer behavior and purchasing decisions. Goal: Higher is better
- Customer Lifetime Value (CLV): AI can help predict the CLV of customers acquired through advertising, allowing advertisers to optimize campaigns for long-term profitability. Goal: Higher is better
Looking Ahead
Applying AI in marketing for ad networks, retailers can improve the effectiveness of their advertising campaigns, drive better engagement with customers, and ultimately increase sales and revenue. Here are some tips for brands looking ahead to build and apply AI in marketing:
- Define Goals: Clearly define your marketing goals and how AI can help achieve them, such as improving ad targeting, increasing conversion rates, or enhancing customer engagement.
- Data Collection and Integration: Collect and integrate relevant data sources, such as customer demographics, behavior, and transactional data, to create a comprehensive view of your customers.
- AI Tool Selection: Choose the right AI tools and platforms for your needs, such as AI-powered ad networks, customer segmentation tools, or predictive analytics platforms.
- Develop AI Models: Develop AI models and algorithms for tasks such as customer segmentation, personalized recommendations, and ad targeting. This may require working with data scientists or AI experts.
- Implement AI in Ad Campaigns: Use AI to optimize ad campaigns by analyzing data, predicting customer behavior, and delivering personalized ads to target audiences.
- Monitor and Analyze Performance: Continuously monitor the performance of your AI-powered ad campaigns using relevant metrics and analytics tools. Analyze the results to identify areas for improvement and optimization.
- Iterate and Refine: Use the insights gained from monitoring and analysis to iterate and refine your AI-powered ad campaigns. Continuously test new ideas and strategies to improve performance.
- Invest in AI Talent: Consider investing in AI talent or partnering with AI experts to help implement and manage your AI-powered marketing initiatives.
- Stay Updated: Keep abreast of the latest trends and advancements in AI and marketing to ensure that your strategies remain relevant and effective.
Working with trusted established ad networks would be a better option than starting a brand new network. The ultimate goal is to establish a better presence in the marketplace to engage, capture and retain customers.
Follow this link for more information about the Retail Mashup content platform
Follow this link for more insights
Follow this link for more podcast episodes
Follow this link to participate in regular polls